Essay: Machine Learning for Factor Investing, by Guillaume Coqueret (PHD 12)
Machine learning (ML) is progressively reshaping the fields of quantitative finance and algorithmic trading. ML tools are increasingly adopted by hedge funds and asset managers, notably for alpha signal generation and stocks selection. The technicality of the subject can make it hard for non-specialists to join the bandwagon, as the jargon and coding requirements may seem out of reach.
This book bridges this gap. It provides a comprehensive tour of modern ML-based investment strategies and covers a wide array of subjects ranging from economic rationales to rigorous portfolio back-testing, data processing and model interpretability. Common supervised learning algorithms such as tree models and neural networks are explained in the context of style investing, and the reader can also dig into more complex techniques like autoencoder asset returns, Bayesian additive trees, and causal models.
All topics are illustrated with self-contained R code samples and snippets that are applied to a large public dataset that contains over 90 predictors. The material, along with the content of the book, is available online so that readers can reproduce and enhance the examples at their convenience. If you have even a basic knowledge of quantitative finance, this combination of theoretical concepts and practical illustrations will help you learn quickly and deepen your financial and technical expertise.
Machine Learning for Factor Investing
Guillaume Coqueret (PHD 12) & Tony Guida
Routledge Publishing
321 pages
£128
Want to read more? Join us so that we can offer you other contents and news about ESSEC alumni.
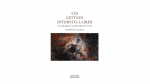
Commentaires0
Vous n'avez pas les droits pour lire ou ajouter un commentaire.
Articles suggérés